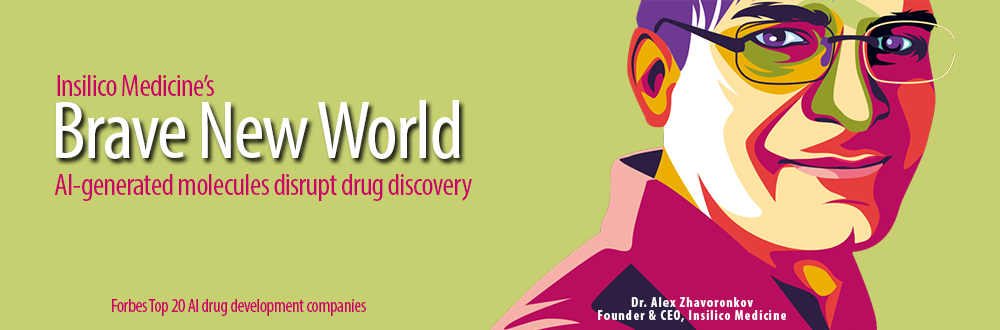
Insilico's Brave New World
AI Goes Where AI Has Never Gone Before
Artificial Intelligence (AI)—in a first-ever—has designed and validated a new drug candidate in days
“Zhavoronkov et al. show that AI techniques can be used to guide our search for good drug molecules in the vastness of chemical space, one of the key challenges in drug discovery today. The work provides compelling evidence that AI can learn from historical datasets to generate novel molecular compounds with drug-like properties, and helps clarify how AI can be used to improve the speed of drug development.”
—Mark DePristo, PhD, former Head of Genomics at Google Brain, Co-founder and CEO, BigHat Biosciences
Margaretta Colangelo
Managing Partner at Deep Knowledge Ventures & Managing Partner at Longevity Capital
Insilico Medicine has succeeded in using AI to
design a new molecule from scratch and
validate it in just 46 days, compared to 2-3
years currently required in the Pharma
industry. This is the first time that the true
transformative and disruptive potential of AI
for the Pharma industry has been validated in
practice, turning its potential from theory into
reality.
HONG KONG
This is Pharma’s AlphaGo moment
The original AlphaGo moment occurred in 2015 when DeepMind succeeded in developing AlphaGo, the first AI capable of beating a human Go champion at Go. This study by Insilico Medicine may be an analogous game-changing moment for Pharma. Insilico Medicine used a generative approach combined with deep reinforcement learning (the form of AI that was used in AlphaGo) to design and validate a new drug candidate end-to-end in 45 days. This is 15x faster than Pharma companies. The experimental validation confirms the ability of AI to accelerate drug discovery.
This landmark study was published in Nature Biotechnology on September 2, 2019. The paper describes a timed challenge, where a new AI system developed by Insilico Medicine called Generative Tensorial Reinforcement Learning (GENTRL) designed six novel inhibitors of DDR1 (a kinase target implicated in fibrosis and other diseases) in 21 days. Four compounds were active in biochemical assays, and two were validated in cell-based assays. One lead candidate was tested and demonstrated favorable pharmacokinetics in mice.
Insilico’s achievement demonstrates the true power of AI to accelerate the pace of scientific R&D. The public is now aware of AI’s potential to transform the Pharma Industry. We expect that this advancement in AI will have a big impact on the Pharma industry generally, and incentivize an increasing number of large Pharma companies to on-board AI in a very integral manner. This news may even create the beginning of an arms race in drug development, where the largest Pharma and Tech corporations compete to acquire the strongest AI in drug discovery companies.
This is the first time that a combination of generative adversarial networks (GANs) and generative reinforcement learning (RL) was used to generate novel small molecules for a protein target that were validated in vitro and in vivo. Insilico was the first company to explore using GANs and RL for drug discovery. Their seminal research led the way for other scientists worldwide to begin developing the theoretical base for GANs and other machine learning techniques to substantially accelerate and improve the drug discovery process.
AI’s potential has been a topic of discussion for years, but so far no AI designed drugs have reached the market. This new study is the closest that the industry has come to demonstrating the real-world potential of AI in drug discovery in a tangible way. This is Insilico’s most important publication to date because it shows that the molecules imagined using the GENTRL approach work in vitro and in vivo. This method could help reduce the 99% preclinical failure rate and to expedite the time it takes to go from R&D to real treatments.
Insilico Medicine is known for validated science – they announce every achievement they make. Insilico has published over 330 scientific papers with over 2,300 citations.
They strive to be transparent in showing that their methodology and their solutions really work. Most AI companies offering similar services barely have a few released papers or a proof of concept derived from their technology. Insilico is known worldwide for being a pioneer in cutting edge science backed up by transparency and credibility. Insilico’s team includes bioinformatics, computational and structural biology experts, highly experienced medicinal chemists, and deep learning engineers, who work with massive amounts of biomedical data and practical problems in drug discovery.
Since 2015 Insilico Medicine has been developing a broad range of GANs and reinforcement learning approaches to identify novel protein targets, generate novel molecular structures with specified properties and generate synthetic data. The company employs over 85 AI experts and scientists in 6 countries and collaborates with over 150 academic and industry partners worldwide. The company is headquartered in HongKong, with R&D and other resources in the US, UK, China, Russia, and Korea.
Insilico is dedicated to transforming the pharma industry by developing and applying next-generation deep learning approaches to every step of the drug discovery and drug development process. The company collaborates with the most innovative biopharmaceutical companies with disease-relevant assays to validate its solutions and generate high-quality machine-learnable data. In addition to working collaborations with the large pharmaceutical companies, the company is pursuing internal drug discovery programs in a range of cancers, fibrosis, CNS diseases, sarcopenia, metabolic diseases, dermatological diseases, and senescence.
"The platforms built on this technology may save millions to tens of millions in R&D costs and 1-2 years in small molecule discovery time. And since the molecules are generated with the specific conditions and objectives in mind including safety, it is likely that these molecules will have better chances of passing through clinical trials.”
Alex Zhavoronkov PhD
Founder & CEO, Insilico Medicine
Deep Knowledge Ventures provided Insilico Medicine’s initial funding in 2014, and has remained a close advisor in the company’s journey towards becoming a global leader in the application of advanced AI for aging research. Insilico Medicine is one of our most promising portfolio companies, not only in terms of its potential ROI, but also of its potential impact on serious problems facing humanity. For over three years Insilico’s scientists have been developing the theoretical base for GANs and other machine learning techniques to substantially accelerate and improve the drug discovery process. Methods like GENTRL could expedite drug discovery dramatically so that life saving treatments could reach doctors and patients sooner, reducing suffering and saving human lives.
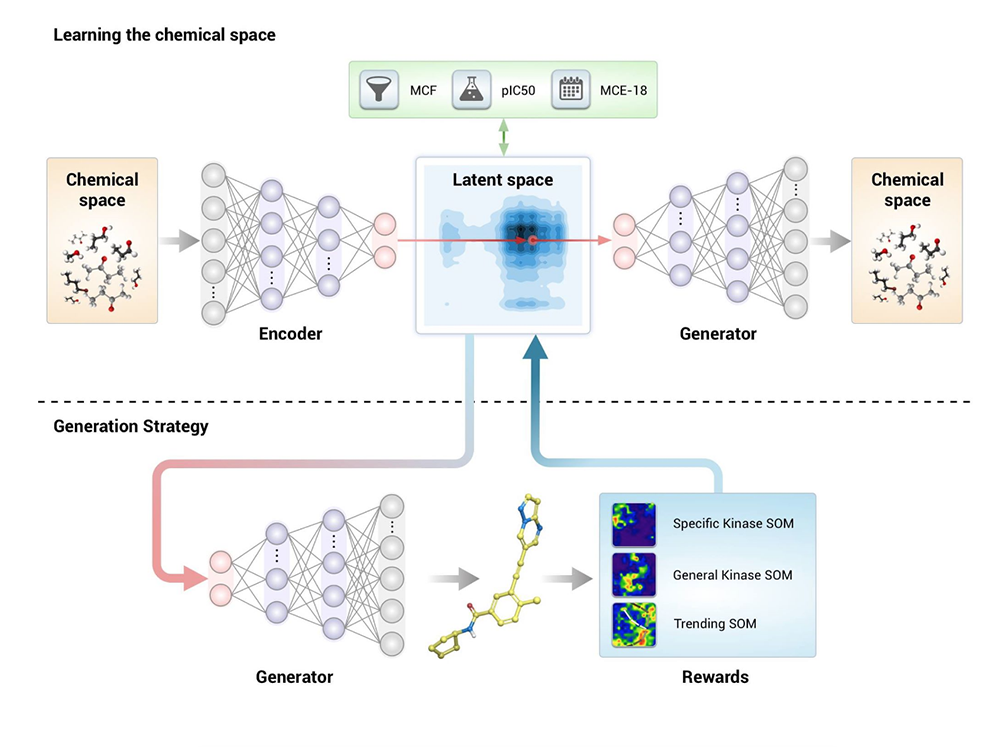
A graphical representation of the GENTRL approach. It generates the molecules with specific conditions and learns to generate molecules with the specific objectives.
From Molecule Design in 2016 to Molecule Validation in 2019
The concept of GANs is relatively new and is sometimes referred to as the AI Imagination. GANs are used to generate images with specific properties. Conceptually it is a competition between two deep neural networks, where one, the generator, is generating novel content with the desired set of criteria, and another, the discriminator, is testing whether the output of the generator is true or false. Insilico Medicine was the first to utilize GANs to generate novel molecules in 2016, and since then has spent two years developing the theoretical base for the combined use of GANs and RL, documented in 15+ papers and 80+ conference presentations.
Insilico Medicine screens potential drug candidates using GANs, which create synthetic datasets that are indistinguishable from real datasets by having two neural networks compete against each other. One neural network generates the data and the other compares it to a real data set in iterative cycles so that the degree of error in the synthetic data set is gradually decreased. Rather than using trial and error when looking for molecular leads, requests are made to the network to generate specific leads and leads are generated on demand.
Applying Deep Learning to Drug Discovery
So, how exactly does this work in practice? The process begins with identification of a protein target. Once a target is identified, scientists use a deep learning algorithm to design molecular structures with desired physical and chemical properties. This is a brand new approach to drug design. The traditional method screens existing molecule libraries against specific targets. Insilico Medicine spent two years developing the theoretical base for the GENTRL method.
Deep generative models are machine learning techniques that use neural networks to produce new data objects. This technique generates objects with specific properties so it’s well-suited to discover drug candidates. This new model optimizes for synthetic feasibility and biological activity. Insilico Medicine performed a challenging experiment where they timed the process from target nomination, small molecule design, synthesis, disease-relevant models, and animal pharmacokinetics for lead-like molecule development.