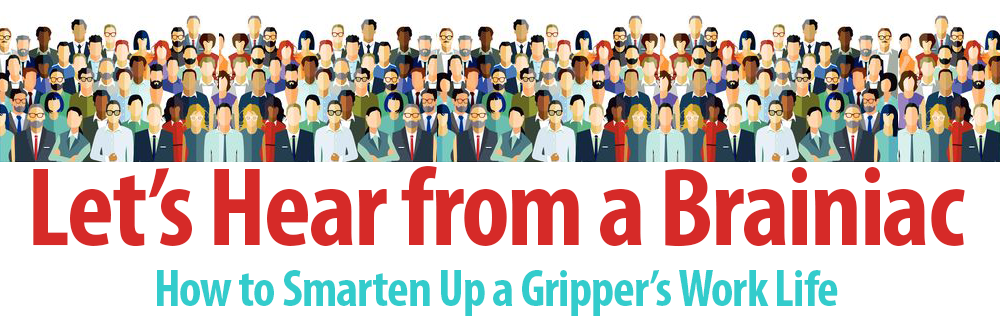
The Gripper Chronicles: Calling All Brainiacs
Building Robot Grippers for ‘General Manipulation’
SHOWCASE: Robotic Materials, Inc. develops robotic grippers with built-in 3D perception, tactile sensing, and computation
At the very least, artificial intelligence (AI) has given us new eyes. These days it’s difficult to look at any mechanical device and not see the potential for some sort of machine intelligence guiding its operation and outcomes.
Five years ago, there might have been less to see in Martin Hägele’s pronouncement that “the end of the arm is where all the action is at.” Five years ago, he might not have been bold enough to say it.
AI has opened a new portal of awareness in robotics labs everywhere, and because of it, all sorts of eureka potential is on the prowl.
If “the end of the arm is where all the action is,” which seems to be the likely outcome for future robots, developers like Nikolaus Correll (founder & CTO) and his mates at Robotic Materials Inc. (Denver, CO) have produced some exciting tech.
Founded in 2016, Robotic Materials, Inc. has raised a total of $225K in funding over 3 rounds. Its latest funding was raised on Dec 1, 2017 from a grant round.
From the website
“Robotic Materials sensors,” explains the company website, “provide the missing link between existing perception systems and (not so) accurate robotic manipulators.
“Proximity allows the robot to make up for inaccuracies of perception and manipulation, contact allows the robot to validate pose without exerting forces, and pressure allows the robot to confirm the object pose relative to its body.”
“Robotic Materials’ autonomous hand fuses 3D perception and tactile sensing to create a high-resolution representation of its environment. Integrated, GPU-enabled perception algorithms provide advanced 3D reasoning and object recognition capabilities that are available to high-level applications like pick-and-place, bin picking, and assembly.”
“We use deep learning for object recognition and 3D perception by combining powerful hand-coded algorithms with self-supervised learning that let the robot adopt to a user’s environment.
“All our applications can be reconfigured using a graphical programming environment, enabling novice programmers to build powerful applications that can be operational the same day you unbox your new robotic hand.”
Where all the action is
Well, all that seems to fit neatly into the Hägelean formula for smart grippers.
Robotic Materials’ brainiac-in-residence, Nikolaus Correll, put together a very interesting guest blog for IEEE Spectrum.
Below are excerpts from the IEEE Spectrumj; the full article can be found here:
Robots Getting a Grip on General Manipulation
By Nikolaus Correll, founder & CTO
From a strictly industrial perspective, ‘general manipulation’ may not even be a problem that is worth solving.
Indeed, you can build a machine for anything, from preparing fantastic espresso, doing your dishes, harvesting a field of wheat, or mass-producing a sneaker. This is how the majority of robots are currently employed in industry.
Even those marketed as “collaborative robots” [cobots] mostly become parts of a sophisticated machine in an automated line (that gets away with less safety caging). Any attempts to develop more generalized manipulation solutions that an academic might be interested in are benchmarked against these use cases.
This makes the advantage of a general solution less obvious, and they risk getting stuck in a valley of inefficiency where investors and industry lose interest. However, manufacturing and delivery processes involve a long tail of highly varying manipulation steps. Even if each step is of negligible value, their cumulative cost is economically significant.
Impedance controlled gripper
Good practical results can be achieved for robotic grippers by combining simple position control with limiting the maximum torque the motors can exert. Like its fully deformable soft-robotic equivalent, an impedance controlled gripper can conform to an object and make up for inaccuracies in perception.
Torque-based sensing at finger joints could be augmented by tactile sensors that measure pressure and are strategically placed across the gripper. Tactile sensors on the palm and the tip might help to differentiate whether finger motion was inhibited by running into an obstacle or by making contact with the object of interest. Tactile sensors also directly complement vision sensors by determining when contact was made, thereby improving object pose estimation, and where in the hand an object was grasped.
Recent advances in 3D perception, however, bring us closer to general manipulation than ever before. 3D sensors such as the Intel RealSense are able to perceive objects as close as 11cm from the camera at accuracies that allow to make out very small items such as M3 screws, and integrated solutions have become commercially available, with my lab’s spin-off Robotic Materials Inc. just releasing a beta version of its hand.
It is the interplay of accurate 3D perception, impedance control for gentle interaction with the environment, and the various ways of tactile sensing to assess grasp success that now can enable robust mobile manipulation in uncertain environments.
Despite their impressive enabling capability for general manipulation, 3D perception, impedance control, and tactile sensing are at odds with the prevailing industrial paradigm of specialized manipulation solutions.
[Therefore], the drivers for ‘general manipulation’ will be SMEs who are working on a large variety of products with small numbers, and larger corporate players who want to differentiate their products with faster production cycles and higher degrees of customization.